Table of Contents
ToggleIntroduction
How Technology Helps Analyze Literary Texts Technology is changing how we approach traditional disciplines, including literature, in the era of digital innovation. Technology is providing new methods for analyzing, comprehending, and interpreting literary works in a variety of ways, from machine learning to artificial intelligence (AI), from digital humanities initiatives to advanced text analysis tools.
For academics and general readers alike, technology has proven to be a priceless tool for deciphering literary style, revealing hidden themes, or offering insights into authorial purpose.
The Role of Technology in Literary Analysis
Literary analysis has long been a labor-intensive, deeply subjective process. Scholars and critics have traditionally relied on close reading, historical context, and their understanding of language and culture to interpret texts. However, as technology has evolved, it has introduced new methods of analysis that offer more precise, efficient, and sometimes entirely new ways to understand literature.
While traditional literary criticism still plays a central role in understanding literature, the integration of technology into literary studies has allowed for greater objectivity, scalability, and depth in analysis. Technology helps uncover patterns and nuances that might otherwise go unnoticed, and it enables readers and researchers to interact with texts in ways that go beyond simple reading. By employing digital tools, it’s now possible to analyze texts from a variety of perspectives: linguistically, syntactically, contextually, and even emotionally.
1. Digital Text Analysis: Tools and Techniques
1.1 Text Mining and Computational Linguistics
Text mining refers to the use of technology to extract meaningful information from large datasets of text. In literature, this involves applying algorithms to analyze patterns, themes, word frequencies, sentence structures, and other linguistic features of a literary work. Researchers use text mining techniques to examine large corpora of literature to identify stylistic elements or linguistic features unique to specific authors, genres, or periods.
Computational linguistics also plays a critical role in text analysis. By applying computational methods to linguistic data, scholars can analyze the syntax and grammar of literary works, studying how authors use language to convey meaning, evoke emotions, or develop characters. Programs can identify key phrases, repetition, metaphors, and even the tone or sentiment of a text, thus providing deeper insights into the underlying themes and structures.
Read More
1.2 Sentiment Analysis
Sentiment analysis, often used in marketing or customer feedback, has found a home in literary studies. By using algorithms to detect the emotional tone behind words, researchers can analyze how different sentiments (anger, joy, sorrow, etc.) are expressed across a literary work. Sentiment analysis tools can break down a text to identify specific moments of emotional intensity and track the emotional arc of characters over time.
For example, a sentiment analysis of Shakespeare’s Hamlet might reveal patterns of melancholy or despair in the character’s speech, offering fresh insights into the emotional depth of his character. These analyses are not only valuable for studying individual texts but can also be used for comparative studies, allowing researchers to study the emotional tones across different works or genres.
1.3 Topic Modeling
Topic modeling is another powerful tool that has emerged from computational linguistics. It involves the use of algorithms to uncover themes or topics that run through a large body of text. Using unsupervised machine learning methods, topic modeling can identify clusters of words that frequently appear together and then group those words into coherent topics.
For instance, topic modeling could be used to study the works of Charles Dickens, revealing how themes such as poverty, social justice, or industrialization are developed across his novels. This method allows scholars to break down complex texts into quantifiable elements, revealing broader thematic structures that might not be immediately apparent through traditional reading.
1.4 Stylometry and Authorship Attribution
Stylometry is the quantitative analysis of writing style. It involves measuring certain features of a text, such as sentence length, word frequency, punctuation use, and other stylistic choices, to identify distinctive writing patterns. This technique can be used to identify authorship, detect plagiarism, or even uncover patterns in an author’s development over time.
In literary studies, stylometry has been employed to solve long-standing authorship debates. For example, by analyzing the stylistic features of the works attributed to William Shakespeare, researchers have attempted to determine whether some of his plays may have been written by other authors. Similarly, stylometric analysis has been used to analyze posthumous works of authors such as J.D. Salinger or Mark Twain.
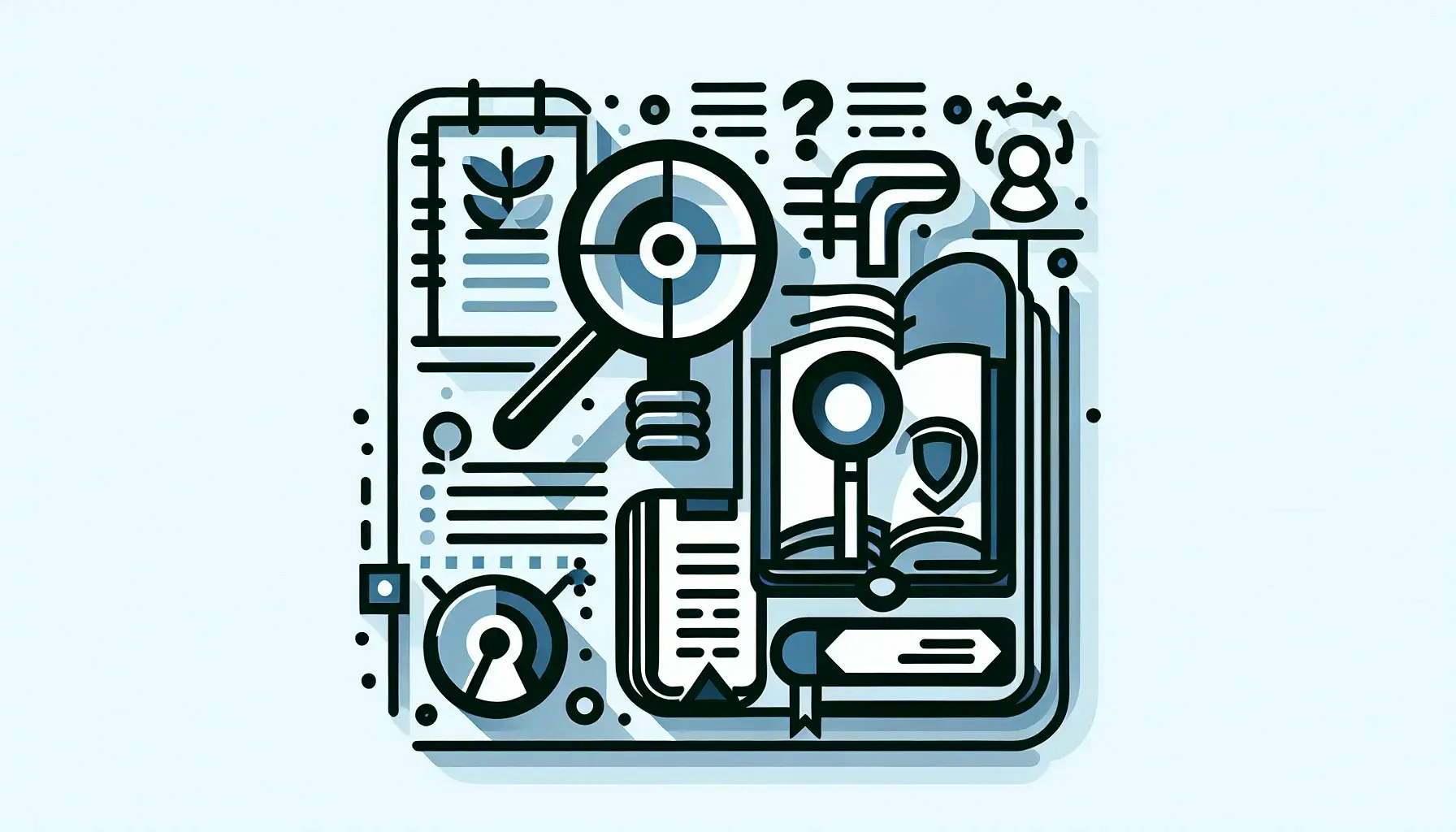
2. Enhancing the Reading Experience with Technology
2.1 E-Readers and Annotating Tools
While e-readers like Amazon’s Kindle and Apple’s iBooks are primarily designed for reading convenience, they also offer valuable tools for literary analysis. Many e-readers come with built-in annotation features that allow readers to highlight, underline, and take notes directly in the text. This enhances the traditional reading experience by providing an interactive layer to engage with the material.
For literature students, the ability to annotate texts digitally and track themes, characters, and motifs across chapters is incredibly useful. Annotating on an e-reader can be a more efficient process than writing notes by hand, allowing for better organization and quick retrieval of insights. Furthermore, digital annotations can be shared with others, opening the door to collaborative analysis and discussion.
2.2 Interactive Texts and Augmented Reality (AR)
In recent years, augmented reality (AR) has been making its way into the literary world. Through AR, readers can interact with books in entirely new ways. For example, AR apps might overlay additional historical context, visual interpretations, or audio commentary on top of the text, enriching the reading experience and offering deeper insights.
Interactive e-books are also gaining popularity, particularly in children’s literature. These books might include interactive features, such as clickable characters or additional multimedia elements, that enrich the reader’s understanding of the text. These innovations are blurring the lines between traditional reading and multimedia storytelling, offering a more immersive literary experience.
3. The Role of Artificial Intelligence (AI) in Literary Analysis
3.1 AI in Text Interpretation
Artificial intelligence, with its natural language processing (NLP) capabilities, is being used to interpret texts in ways that were not possible before. By feeding AI algorithms vast amounts of literary data, these systems can learn to identify literary devices, interpret metaphors, and analyze complex narratives. AI can detect narrative patterns, trace character arcs, and even predict the development of a plot based on existing narrative structures.
For example, AI can be used to analyze the character development of an epic novel, such as Moby-Dick, identifying how Ishmael’s thoughts and actions evolve over time. These systems can then compare these developments to similar character journeys in other novels, identifying literary patterns or genre conventions.
3.2 AI-Driven Literary Criticism
AI is also being used to assist in literary criticism, especially in the analysis of recurring themes or symbols. Machine learning algorithms can be trained to recognize recurring imagery, themes, and motifs across different genres or time periods. This could offer new insights into how certain symbols have evolved over time, such as the image of the “forest” in Gothic literature or the symbol of the “moon” in Romantic poetry.
AI-driven literary criticism can help bridge the gap between traditional critical approaches and contemporary technological methods, providing a comprehensive analysis of texts that combines human intuition and machine precision.
3.3 Chatbots and Virtual Literary Conversations
Some innovative platforms are utilizing AI-powered chatbots to create virtual literary discussion groups. These bots can engage in conversations about books, analyze the plot, or offer personalized recommendations based on the reader’s preferences. These AI systems can simulate a literary discussion by answering questions, offering interpretations, or providing analysis of specific passages, creating a unique way to engage with literature outside of traditional reading circles.
Read More
4. Ethical Considerations and Challenges in Literary Text Analysis
While the integration of technology into literary studies offers many exciting opportunities, it also raises important ethical questions and challenges. For instance, the use of AI and machine learning algorithms to analyze literary works may result in biases, as these systems often reflect the biases inherent in the data they are trained on. Additionally, AI’s ability to analyze text may inadvertently overshadow the nuances of human interpretation, reducing the richness of subjective readings in favor of data-driven conclusions.
Another challenge lies in ensuring that technology does not replace human expertise. While AI can assist in identifying patterns or offering new insights, human readers are still essential for providing cultural and emotional context that machines cannot fully grasp.
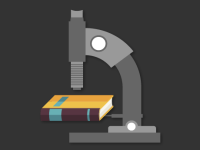
Conclusion
Technology is transforming the landscape of literary analysis in profound ways. From AI-driven tools that uncover hidden themes to computational linguistics that help us understand authorial intent, the role of technology in literature has never been more significant. By blending human creativity with technological precision, we can gain deeper insights into literary texts, enhancing our appreciation of the art of storytelling. As these technologies continue to evolve, they will undoubtedly offer even more exciting possibilities for the future of literary analysis.
Read More
FAQ
1. What is text mining in literary analysis?
Text mining involves using computational techniques to extract patterns, themes, and other meaningful data from large bodies of text. In literary studies, text mining can reveal stylistic patterns, thematic trends, and linguistic structures that may not be immediately evident from a traditional reading.
2. How does sentiment analysis work in literature?
Sentiment analysis uses algorithms to analyze the emotional tone behind the words in a text. It identifies emotions like joy, sadness, or anger, and can track the emotional evolution of a character or scene throughout a literary work.
3. Can AI replace human literary critics?
While AI can assist with text analysis by identifying patterns and generating insights, it cannot fully replace human literary critics. AI lacks the cultural, emotional, and historical context that human critics bring to their interpretations, making the combination of AI and human expertise more effective.
4. What are the benefits of digital annotations in literary analysis?
Digital annotations allow readers to highlight, underline, and note key passages directly within a text, making it easier to organize thoughts and track important themes. These annotations can be saved, shared, and revisited, offering a more efficient way to engage with complex literary works.
5. What ethical concerns arise from using AI in literary analysis?
AI-based literary analysis raises concerns about bias, as algorithms may reflect the prejudices present in the data they are trained on. Additionally, there is the risk of AI overshadowing human interpretations by focusing on data-driven conclusions rather than the subjective and cultural insights that human critics provide.